bagging resampling vs replicate rsampling | bagging bagging resampling vs replicate rsampling First, definitorial answer: Since "bagging" means "bootstrap aggregation", you have to bootstrap, which is defined as sampling with replacement. Second, more interesting: Averaging predictors only improves the prediction if they are not overly correlated. The replacement reduces similarity of data, and hence correlation of predictions.
We would like to show you a description here but the site won’t allow us.
0 · bagging
1 · What is Bagging in Machine Learning? A Guide With Examples
2 · How to Create a Bagging Ensemble of Deep Learning Models in
3 · How is bagging different from cross
4 · Hierarchical resampling for bagging in multistudy prediction with
5 · Ensemble methods: bagging and random forests
6 · Comparing Boosting and Bagging for Decision Trees of Rankings
7 · Bootstrapping and Bagging: Enhancing Predictive Modeling
8 · Bagging and Boosting
9 · Bagging
2015. Preview. In the time that’s passed since 2007’s Christmas-themed And Winter Came, Enya’s influence has made itself heard in a new generation of female artists—from Julia Holter’s classically informed art-pop to the hallucinogenic melodies of Grimes.
bagging
The big difference between bagging and validation techniques is that bagging averages models (or predictions of an ensemble of models) in order to reduce the variance the prediction is subject to while resampling validation such as cross validation and out-of-bootstrap validation evaluate a number of surrogate models assuming that they are . The central idea behind bootstrapping is resampling: by drawing repeated samples from the observed data with replacement, statisticians and data scientists can estimate the sampling distribution of a statistic without relying on strong distributional assumptions. The key components of bootstrapping include. We briefly outline the main difference between bagging and boosting, the ensemble methods we are going to work with. Bagging (Section 4.1) learns decision trees for many datasets of the same size, randomly drawn with replacement from the training set. Thereafter, a proper predicted ranking is assigned to each unit. Perhaps the most widely used resampling ensemble method is bootstrap aggregation, more commonly referred to as bagging. The resampling with replacement allows more difference in the training dataset, biasing the model and, in turn, resulting in more difference between the predictions of the resulting models.
The bagging technique is a useful tool in machine learning applications to improve model accuracy and stability. Learn ensemble techniques such as bagging, boosting, and stacking to build advanced and effective machine learning models in Python with the Ensemble Methods in Python course. First, definitorial answer: Since "bagging" means "bootstrap aggregation", you have to bootstrap, which is defined as sampling with replacement. Second, more interesting: Averaging predictors only improves the prediction if they are not overly correlated. The replacement reduces similarity of data, and hence correlation of predictions.
omega seamaster.
We term such collection a “study strap replicate” and each member a “pseudo-study.” We refer to the original studies, without any resampling, as “observed studies” and the resampling procedure as the “study strap.” Each pseudo-study can then be used as a training dataset to fit a prediction model. Bagging is a common ensemble method that uses bootstrap sampling 3. Random forest is an enhancement of bagging that can improve variable selection. We will start by explaining bagging and then. To approximate a limitless number of independently realized datasets, a large number of probability samples are drawn with replacement from the single realized dataset; hence the term “resampling.”. These probability samples are denoted by \ ( b_ {1}, b_ {2} \ldots , b_ {B}\), where B is the total number of samples.The idea is of adaptively resampling the data • Maintain a probability distribution over training set; • Generate a sequence of classifiers in which the “next” classifier focuses on sample where the “previous” clas sifier failed; • Weigh machines according to their performance.
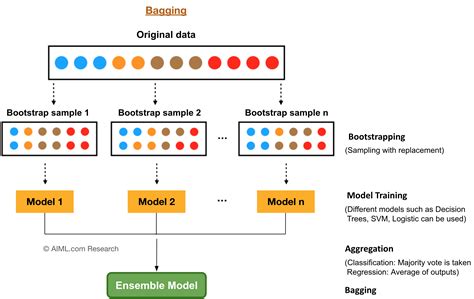
The big difference between bagging and validation techniques is that bagging averages models (or predictions of an ensemble of models) in order to reduce the variance the prediction is subject to while resampling validation such as cross validation and out-of-bootstrap validation evaluate a number of surrogate models assuming that they are . The central idea behind bootstrapping is resampling: by drawing repeated samples from the observed data with replacement, statisticians and data scientists can estimate the sampling distribution of a statistic without relying on strong distributional assumptions. The key components of bootstrapping include.
We briefly outline the main difference between bagging and boosting, the ensemble methods we are going to work with. Bagging (Section 4.1) learns decision trees for many datasets of the same size, randomly drawn with replacement from the training set. Thereafter, a proper predicted ranking is assigned to each unit. Perhaps the most widely used resampling ensemble method is bootstrap aggregation, more commonly referred to as bagging. The resampling with replacement allows more difference in the training dataset, biasing the model and, in turn, resulting in more difference between the predictions of the resulting models. The bagging technique is a useful tool in machine learning applications to improve model accuracy and stability. Learn ensemble techniques such as bagging, boosting, and stacking to build advanced and effective machine learning models in Python with the Ensemble Methods in Python course. First, definitorial answer: Since "bagging" means "bootstrap aggregation", you have to bootstrap, which is defined as sampling with replacement. Second, more interesting: Averaging predictors only improves the prediction if they are not overly correlated. The replacement reduces similarity of data, and hence correlation of predictions.
We term such collection a “study strap replicate” and each member a “pseudo-study.” We refer to the original studies, without any resampling, as “observed studies” and the resampling procedure as the “study strap.” Each pseudo-study can then be used as a training dataset to fit a prediction model.
Bagging is a common ensemble method that uses bootstrap sampling 3. Random forest is an enhancement of bagging that can improve variable selection. We will start by explaining bagging and then.
To approximate a limitless number of independently realized datasets, a large number of probability samples are drawn with replacement from the single realized dataset; hence the term “resampling.”. These probability samples are denoted by \ ( b_ {1}, b_ {2} \ldots , b_ {B}\), where B is the total number of samples.The idea is of adaptively resampling the data • Maintain a probability distribution over training set; • Generate a sequence of classifiers in which the “next” classifier focuses on sample where the “previous” clas sifier failed; • Weigh machines according to their performance. The big difference between bagging and validation techniques is that bagging averages models (or predictions of an ensemble of models) in order to reduce the variance the prediction is subject to while resampling validation such as cross validation and out-of-bootstrap validation evaluate a number of surrogate models assuming that they are .
What is Bagging in Machine Learning? A Guide With Examples
The central idea behind bootstrapping is resampling: by drawing repeated samples from the observed data with replacement, statisticians and data scientists can estimate the sampling distribution of a statistic without relying on strong distributional assumptions. The key components of bootstrapping include. We briefly outline the main difference between bagging and boosting, the ensemble methods we are going to work with. Bagging (Section 4.1) learns decision trees for many datasets of the same size, randomly drawn with replacement from the training set. Thereafter, a proper predicted ranking is assigned to each unit.
Perhaps the most widely used resampling ensemble method is bootstrap aggregation, more commonly referred to as bagging. The resampling with replacement allows more difference in the training dataset, biasing the model and, in turn, resulting in more difference between the predictions of the resulting models. The bagging technique is a useful tool in machine learning applications to improve model accuracy and stability. Learn ensemble techniques such as bagging, boosting, and stacking to build advanced and effective machine learning models in Python with the Ensemble Methods in Python course. First, definitorial answer: Since "bagging" means "bootstrap aggregation", you have to bootstrap, which is defined as sampling with replacement. Second, more interesting: Averaging predictors only improves the prediction if they are not overly correlated. The replacement reduces similarity of data, and hence correlation of predictions. We term such collection a “study strap replicate” and each member a “pseudo-study.” We refer to the original studies, without any resampling, as “observed studies” and the resampling procedure as the “study strap.” Each pseudo-study can then be used as a training dataset to fit a prediction model.
Bagging is a common ensemble method that uses bootstrap sampling 3. Random forest is an enhancement of bagging that can improve variable selection. We will start by explaining bagging and then. To approximate a limitless number of independently realized datasets, a large number of probability samples are drawn with replacement from the single realized dataset; hence the term “resampling.”. These probability samples are denoted by \ ( b_ {1}, b_ {2} \ldots , b_ {B}\), where B is the total number of samples.
How to Create a Bagging Ensemble of Deep Learning Models in
How is bagging different from cross

Discover Louis Vuitton Palm Springs Mini: The Palm Springs Mini gives a fresh twist to the backpack, transforming a utilitarian staple into an on-point city bag. An essential accessory for sporty urban nomads, this charming model is crafted from Monogram canvas with soft leather trim and gold-tone hardware. The quilted, foam-backed straps can be .
bagging resampling vs replicate rsampling|bagging